By shamvi
In
2022-10-112023-02-15https://rhed.amsi.org.au/wp-content/uploads/sites/73/2020/06/amsi_rhed_v2-2.pngResearch and Higher Education
200px200px
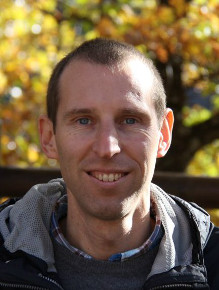
The AMSI-ANZIAM Lecture Tour invites a distinguished international academic in an applied mathematical field to speak at universities across Australia after the conclusion of the ANZIAM conference. It includes a series of talks including specialist and public lectures. The tour is organised biennially by AMSI and is supported by ANZIAM.
Professor Konstantin Avrachenkov
National Institute for Research in Digital Science and Technology (INRIA)
Konstantin Avrachenkov received his Master degree in Control Theory from St. Petersburg State Polytechnic University (1996), Ph.D. degree in Mathematics from University of South Australia (2000) and Habilitation from University of Nice Sophia Antipolis (2010). Currently, he is a Director of Research at Inria Sophia Antipolis, France. He is an associate editor of the International Journal of Performance Evaluation, Probability in the Engineering and Informational Sciences, ACM TOMPECS, Stochastic Models and IEEE Network Magazine. Konstantin has co-authored two books “Analytic Perturbation Theory and its Applications”, SIAM, 2013 and “Statistical Analysis of Networks”, Now Publishers, 2022. He has won 5 best paper awards. His main theoretical research interests are Markov chains, Markov decision processes, random graphs and singular perturbations. He applies these methodological tools to the modeling and control of networks, and to design data mining and machine learning algorithms.
Prior to the lecture tour, Professor Konstantin Avrachenkov will deliver a plenary talk at the ANZIAM Conference held in Cairns from 5-9 February 2023.
PODCAST: Recorded at the recent ANZIAM Conference, Professor Avrachenkov caught up with The Random Sample to feature in their latest episode. LISTEN NOW
Date | Time* | Type | TITLE | HOST | Venue/Location | Watch Online |
---|---|---|---|---|---|---|
Monday 13 February | 11am - 12pm ACDT | Specialist | Singularly Perturbed Markovian Models: From Queues to Web Ranking and Reinforcement Learning | University of South Australia | Room F1-24 University of South Australia, Mawson Lakes campus Adelaide, SA | |
Wednesday 15 February | 11am - 12pm AEDT | Specialist | Random-walk Based Sampling in Social Networks | RMIT | Access Grid Room, Level 3, Building 15, RMIT Melbourne Campus Melbourne, VIC and online | |
Friday 17 February | 11am AEDT | Specialist | Random Graph Models, Network Centralities and Graph Clustering | Australian Bureau of Statistics | Knibbs Theatre, Ground floor, ABS House, 45 Benjamin Way, Belconnen Canberra, ACT | |
Monday 20 February | 2pm AEDT | Specialist | Reinforcement Learning for Restless Bandits | University of Newcastle | Newcastle, NSW | View lecture recording |
Wednesday 22 February | 4pm-5.30pm AEST | Public | Aesthetics and ubiquitous applications of Markov chains | The University of Queensland | Building 7 (Parnell) room 222, Saint Lucia Brisbane, QLD | View lecture recording |
Monday 13 February, University of South Australia
Specialist Lecture: Singularly Perturbed Markovian Models: From Queues to Web Ranking and Reinforcement Learning
Markov chains represent a versatile tool for modelling phenomena in nature and technology. Many phenomena unfold on several time scales. In this talk I first give an accessible introduction to Markov chains and in particular to singularly perturbed Markov chains, which are stochastic dynamical models with several time scales. Then, I demonstrate the application of singularly perturbed Markov chains to queueing systems, web ranking and reinforcement learning.
Wednesday 15 February, RMIT
Specialist Lecture: Random-walk Based Sampling in Social Networks
How many friends do social network members have on average? What is a proportion of a certain sub-population in a social network? Are online social network users more likely to form friendships with those with similar attributes? Such questions frequently arise in the context of social network analysis, but often crawling an online social network via its application programming interface and conducting surveys in offline social networks are resource consuming and are prone to errors. Using regenerative properties of the random walks, we describe estimation techniques based on short crawls that have proven statistical guarantees. Moreover, these techniques can be implemented in low-complexity distributed algorithms.
Friday 17 February, Australian Bureau of Statistics
Specialist Lecture: Random Graph Models, Network Centralities and Graph Clustering
Many real-world complex networks share a number of common properties such as sparsity, heavy-tailed degree distribution, the existence of a giant connected component, small world property and edge transitivity. Firstly, I review several basic random graph models such as Erdos-Renyi random graph, exponential family of random graph models (ERGMs), stochastic block models (SBMs), random geometric graphs, and indicate which model can represent well a given property. Secondly, I describe the main network centrality indices which can be applied to study network structure or to assess network robustness. I conclude with an overview of main methods in graph clustering with a particular emphasis on the methods designed with the help of random graph models and on the methods using centrality indices.
Monday 20 February, University of Newcastle
Specialist Lecture: Reinforcement Learning for Restless Bandits
The Whittle index policy is a heuristic that has shown remarkably good performance and guaranteed asymptotic optimality when applied to the class of hard problems known as Restless Multi-Armed Bandit Problems (RMABPs). Some examples of applications of RMABPs are: machine maintenance, wireless channel scheduling, A/B testing and clinical trials, just to name a few. RMABP provides a classical example when a decision-maker needs to balance between exploration and exploitation. We present two approaches (tabular and neural network based) for learning the Whittle indices. The key feature of our approaches is the usage of two time-scales, a faster one to update the state-action Q-values, and a relatively slower one to update the Whittle indices. The neural network based approach computes the Q-values on the faster time-scale and is able to extrapolate information from one state to another, which makes the approach naturally scalable to environments with large state spaces. We present both the theoretical convergence analysis as well as illustrations by numerical examples.
Wednesday 22 February, The University of Queensland
Public Lecture: Aesthetics and ubiquitous applications of Markov chains
Markov chains, mathematical models that describe sequences of dependent events, were created to make a point in a philosophical discussion and to explain the beauty of the poetry. Even though we may debate the practicality of explanations of aesthetics, it is generally accepted that Andrey Markov (1856-1922) contributed to this philosophical dispute and, in the process, originated one of the most powerful tools of applied mathematics, physics and data science.
In this talk, I first give an accessible introduction to Markov chains and in particular to singularly perturbed Markov chains. These are stochastic dynamical models with several time scales and, as such, are well suited to represent many natural and technological phenomena. In particular, I discuss the application of Markov chains and singularly perturbed Markov chains in linguistics, linked data analysis and reinforcement learning.
The Random Sample caught up with Prof Avrachenkov at the recently held ANZIAM 2023 Conference in Cairns. Also joining us for this episode is his PhD Supervisor from some 20 years ago, Emeritus Professor Jerzy Filar.
In this podcast, we explore the field of mathematics from an international perspective that both of these men bring.